Sports Math: Going Streaking?
“Feeling it!” “On a roll!” “In a funk!” Anyone who follows sports has heard these clichés throughout the course of a season. The implication is that if the going is good, it will continue, and if it isn’t, it’s hard to break that cycle. It’s the elusive concept of momentum. But is it real?
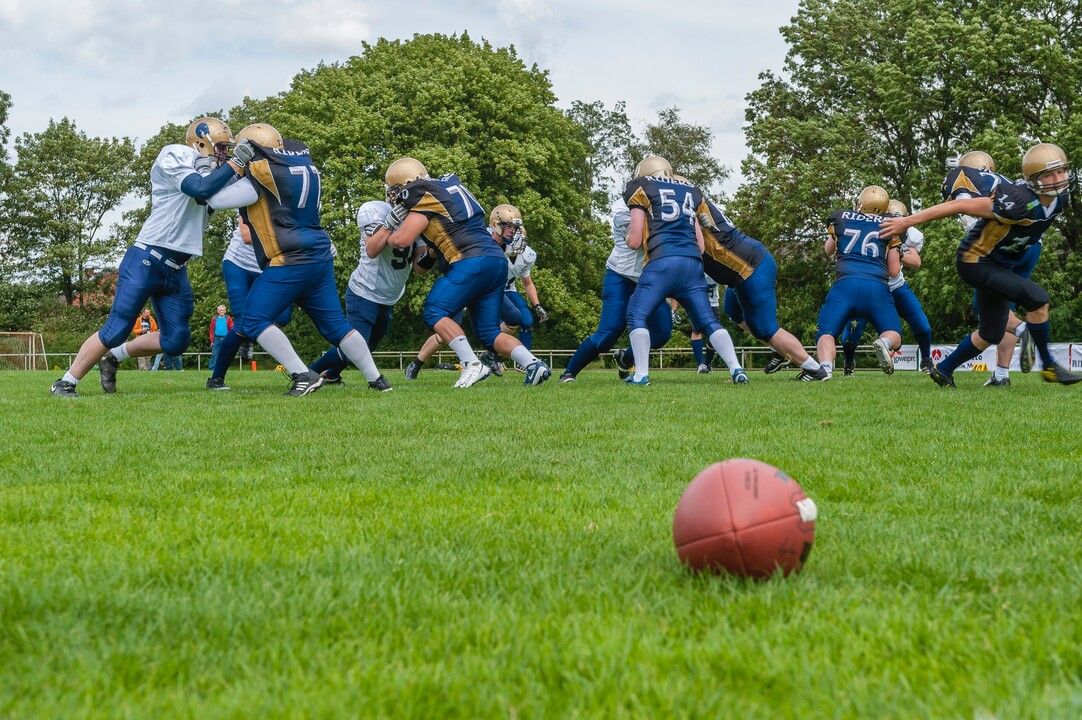
“Feeling it!” “On a roll!” “In a funk!” “Going through a rough patch!” “Hot (or cold) streak!” Anyone who follows sports with any detail has heard these clichés from players or coaches throughout the course of a season. The implication seems to be that if the going is good, it will continue, and if it isn’t, it’s hard to break that cycle. It’s the elusive concept of momentum. But is it real? In this article, we’ll try to answer that question.
First of all, I will find a way to define momentum. This is by no means the only way to define momentum, but it’s something relatively simple that we can start with.
Classroom Connection: If you're looking for a way to connect mathematics to sports and engage students who may not always be interested in math, stats is a great way to do this. Have your students define and create their own statistic for their favorite sport or game. This is a great low floor, high ceiling problem that each student can make their own and take as far as they would like. Some stats are relatively simple to calculate, like number of goals or number of wins, while others are incredibly complex, like the various different definitions of WAR (Wins Above Replacement) in baseball. The website Sports Reference has a huge amount of free data that might provide some inspiration
Have your students define and create their own statistic for their favorite sport or game.
But back to the problem at hand, I will define momentum around the idea that, if a team won their previous game, they are more likely to win their next game, and if they lost their last game, they are more likely to lose their next game, compared to their overall chances of winning or losing a game. If momentum is real, once a team has won a game, it should be more likely that they win another one, and if they lose, they should be more likely to lose the next one. If momentum is real, teams should go on winning or losing streaks more often than would generally be expected.
To understand how momentum might look, consider some cases. I’ll give sample records for a few different teams here:
- Team A: WWWWWWWWWW
- Team B: WWWWWLLLLL
- Team C: LWLWLWLWLW
- Team D: LWLWWWLWLLW
First, let’s look at Team A. Every one of their wins followed a win in a previous game, but I’m loath to say that this is the result of momentum. Maybe it’s just because this team is just really good. After all, they won all of their games. So whatever score for momentum I create, I don’t want to score Team A too high.
Team B and Team C are far more interesting. Both teams have a winning percentage of 0.500, winning 5 out of 10 of their games, but they’ve gone about it in very different ways. Team B started off on fire, winning their first 5 games, but once they lost a game, they didn’t win anything for the rest of the year. Team C, however, alternated losses and wins all season. Whatever our score is for momentum, we should expect Team B to score high, and Team C to score low.
Team D is an example that is somewhere in the middle.
Classroom Connection: This exercise of "What would we expect the score to be?" is going to be important when we are assessing the validity of a created statistic. If we create a statistic that supposedly defines a "good" player for instance, but it consistently rates LeBron James, Diana Taurasi, Serena Williams or Connor McDavid as mediocre or poor, then it's probably worth re-thinking our statistic. While you don't want to create a statistic just so it will show you what you already know, it's also important that it passes a sanity test. Encourage your students to think critically about whether or not their statistic returns data that makes sense.
Encourage your students to think critically about whether or not their statistic returns data that makes sense.
Momentum Calculation Example
Here’s how I chose to define a Momentum Score.
Step 1: Calculate the winning percentage of a team across all the games in the season (number of wins divided by number of games played)
Step 2: Determine the number of wins that immediately followed another win. In the above examples, Team A has 9 of these wins, Team B has 4 (Games 2, 3, 4 and 5), Team C has none and Team D has 2 (Games 5 and 6). These are the "Wins after Wins"
Step 3: Divide the number of these wins after wins by the total number of wins. I've called this a "Wins after Wins percentage" or "W after W %"
Note that if a team wins the first game of the year, that game by definition cannot follow another another win. So we’ll discount the first game of the year as one of the wins, as it couldn’t fall after a win.
- For Team A, 9 out of 9 wins that could follow a win did (1.000).
- For Team B, 4 out of 4 wins that could have come after a win, did (the first win was in the first game) (1.000)
- For Team C, 0 out of 5 wins followed another win. (0.000)
- For Team D, 2 out of 5 wins followed another win. (0.400)
Step 4: Subtract the win percentage from step 1 from the percentage in step 3 to calculate the momentum score.
- A score greater than 0 means a team is more likely to win after winning the previous game
- A score of 0 means that the result of a previous game doesn’t have any impact on a team’s ability to win
- A score less than 0 means that teams are more likely to lose a game after winning the previous one.
If momentum is a real thing, then we should expect this momentum score to be positive, as wins will beget more wins.
So let’s test this on some real data, and see what happens!
A big thank you to https://www.sports-reference.com/ for the raw data that I used for this analysis.
I pulled the results of every single game in the NFL and the NHL for the last three completed seasons, and looked calculated the momentum scores for each team in each season. See the data here.
Conclusions
Conclusion #1: Overall, the effects of momentum seem to be negligible.
Across three years of data, the average momentum score for both the NHL and the NFL was -0.002.
In both leagues, on average slightly less than half of the teams ended up with a positive momentum score. That is, for most teams, the chances of winning their next game actually decreased slightly if they won their previous game. And the middle 50% of the scores fell between approximately 0.02 and -0.04 for the NHL, and 0.06 and -0.06 for the NFL. This means that for most teams, they were only 2-6% more or less likely to win their next game depending on the results of their previous one.
Conclusion #2: A longer season leads to less of an impact in terms of momentum.
Because of how I defined momentum, one long winning streak will have a much larger impact in terms of the overall momentum score of a season if fewer games have been played. For example, a four game winning streak in the NFL counts for 25% of the season, which would be equivalent to a 20 game win streak in the NHL. For that reason, the momentum scores in the NFL were more spread out than the NHL, with a larger range, larger maximum scores and lower minimum scores.
Conclusion #3: There seems to be one outlying team… the Detroit Red Wings.
While some teams may be more heavily influenced by momentum in one given year, like the San Jose Sharks in 2019-2020, the Detroit Red Wings of the NHL have three of the top five momentum scores over the past 3 years. Their average momentum score of over twice as high (0.131) as the average momentum score of any other team in the NHL, and no other team in either league appears more than once in their own league’s top 10 momentum scores.
Conclusion #4: Overall, “good” or “bad” teams aren’t any more or less susceptible to momentum
I was curious about whether or not good teams would be better able to harness the power of the streak, or whether bad teams would fall victim to more losing streaks. In both cases, the answer seems to be no.
While looking at the top 10 momentum scores above indicates shows more bad teams with higher momentum scores, the Pearson’s correlation coefficient between a team’s winning percentage and their momentum score is close to 0 in both cases (-0.099 for the NHL and -0.154 for the NFL). There is a slight tendency for bad teams to have a higher winning percentage, but it’s a weak correlation.
The graphs below show a fairly randomly distributed scatterplot of data, with no obvious patterns.
Classroom Connection: Depending on what kind of statistic your students have defined, this is a great time to get them to use data visualizations to help explain their data. They can discuss the suitability of one type of visualization over another, depending on what their data shows. It also highlights that mathematics is more than just equations and numbers, and can make the content more accessible to students who consider themselves to be more visual as well.
Mathematics is more than just equations and numbers, and can make the content more accessible to students who consider themselves to be more visual as well.