Revolutionizing Mathematics Assessments Pt. 2: Validation through Personalization
The second of a four-part series looking at how Mathspace Check-Ins work, why they matter and how to implement them effectively. Pt. 2 provides an in-depth explanation of how we ensure accuracy in assessment.
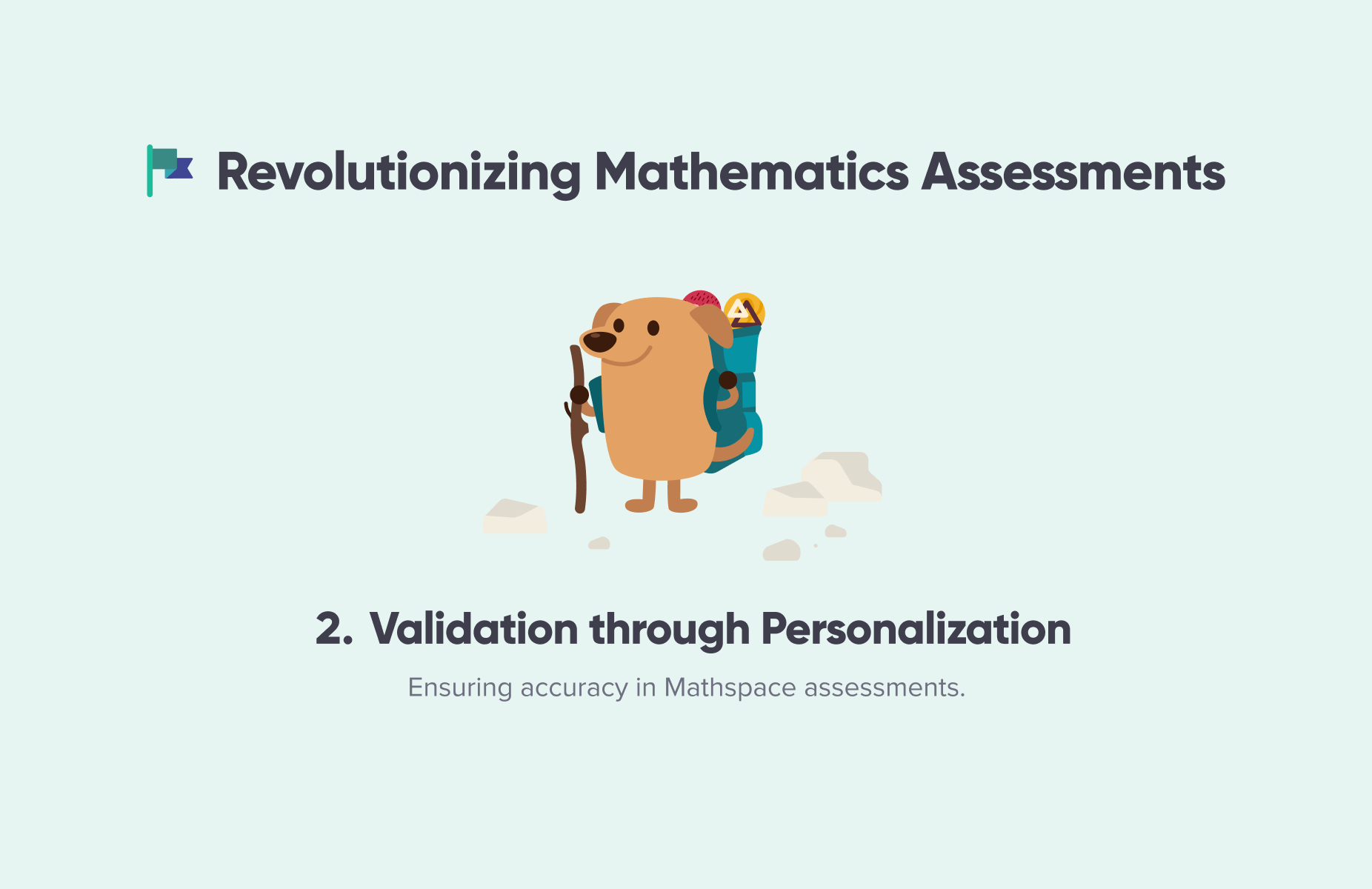
In the first post of this series, we looked at how Check-Ins assess competencies. We looked first at how the Discovery phase generates a baseline for student knowledge and understanding. Then we covered how the Growth phase builds on that baseline with regular Check-Ins to maintain accuracy over time.
In this post, we'll move on to exploring how the format of Check-Ins ensures accuracy in assessment, regardless of a student's starting point.
How we address individuality
A core feature of Check-Ins is that they are adaptive. This adaptivity allows the assessment to be both more efficient and more effective at identifying which questions to ask, and therefore at identifying competencies.
Check-Ins attribute a student's performance on each question to that student’s individual knowledge graph. The knowledge graph specifies how skills are connected so that as students answer questions their proficiency for the tested skill and related skills is updated in real-time.
In addition to the measure of a student's understanding of a skill, the knowledge graph also assigns a score to the confidence we have in that measure. That confidence score is partially determined by the quantity of questions a student has answered on a skill or related skills. Additionally, the score is impacted by the quality of the questions i.e. how accurately specific questions have been at predicting understanding for other students.
Our algorithm then uses all this data to identify the best question to present next. The question it selects is the one that will provide the greatest increase in the confidence score.
In the Discovery phase, we can choose a best question from any skill in the strand (e.g. number) but only up to one grade-level above the student’s specified grade. However, in the Growth phase, we are limited to a substrand (e.g. fractions), but across all grade levels.

One of the most complex aspects of assessment is ensuring validity. In other words, are the assessments truly measuring what they're intended to measure? Because Check-Ins are adaptive (as described above), the assessment mirrors each student’s learning path and is tailored to their level. In addition to this, the questions used in the Check-Ins are carefully designed to maximise the likelihood that a correct response is due to an understanding of the underlying concept rather than a lucky guess.
The format of Check-Ins as continuous assessments, where each Check-In adds to the data generated by prior assessments, is also key to their success. This format, along with the careful question design and adaptive personalisation, ensures that over time the likelihood of validity continues to increase. In contrast, traditional high-stakes assessment starts from scratch each time.
The final piece of the puzzle is our continued work to assess and improve our knowledge graph. We periodically run and analyse offline models using machine learning to fine-tune the knowledge graph and relative question difficulties.
In the next post in this series, we take a look at how we make use of the data generated by Check-Ins to take action.
If you would like to explore Check-Ins for yourself, you can sign up for a free student or teacher account or try our demo Check-In.